High-quality data allows companies to make smart, informed decisions based on accurate insights. However, poor data quality continues to plague many businesses, leading to flawed analysis, costly mistakes, and lost opportunities. What causes data quality issues, and how can organizations address them?
Common Culprits of Bad Data
There are many potential root causes of poor data quality, including:
Lack of Data Governance: Without formal data governance policies, standards, and ownership in place, data quality has no accountability. Different departments or individuals may have their own processes, tools, or databases, leading to fragmented and inconsistent data across the organization.
Human Error: When data is entered manually, typos, accidentally skipping fields, or inconsistent formatting can lead to data quality problems. Poor training on data entry procedures exacerbates this issue.
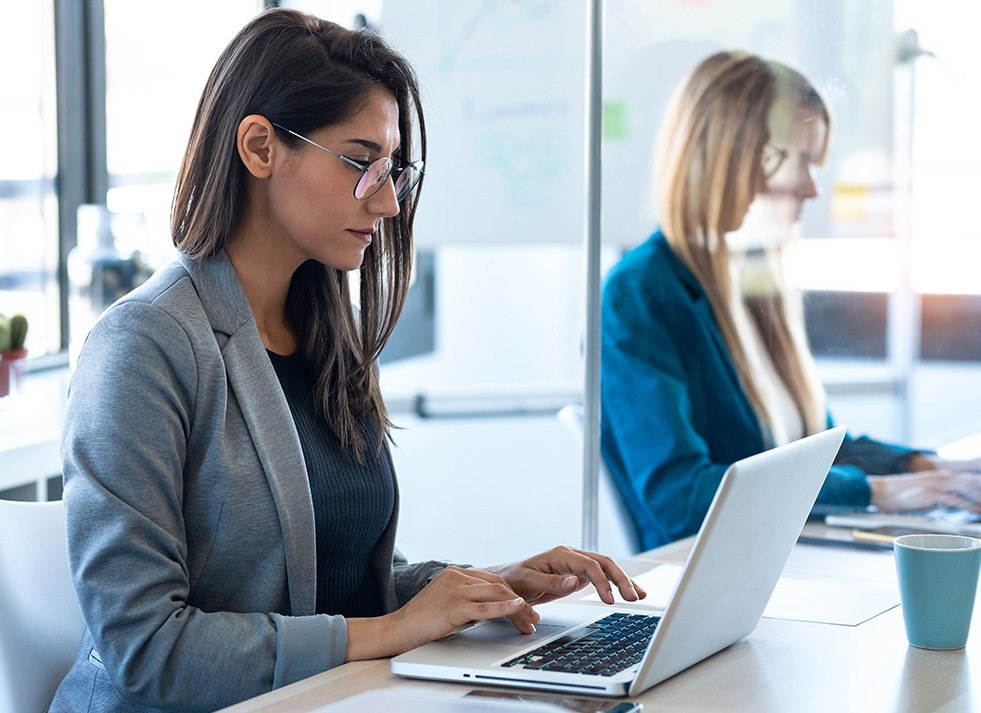
System Issues: If different systems use different formats, fields, or definitions for the same data points, it creates conflicting data when combined. Lack of data validation rules also allows bad data to sneak through. For instance, if a CRM system lacks proper validation for email addresses, it may accept invalid or misspelled email addresses without raising any warnings. This can result in a database filled with incorrect email addresses, making it difficult to reach customers effectively. Without validation checks, other types of errors such as incorrect phone numbers, addresses, or payment information can also be stored in the CRM system, compromising its overall data quality.
Process Flaws: Lack of clear processes around data collection, entry, integration, and maintenance can lead to data hygiene issues. For example, consider the recording of customer addresses. If one employee enters “Street” as “St,” while another employee uses “Str,” and yet another employee spells it out as “Street,” it creates inconsistency and confusion. When conducting targeted marketing or generating customer reports based on address data, this inconsistency can lead to inaccurate segmentation or incomplete analysis. Consistent practices, such as using standardized abbreviations and formats, are essential for maintaining high-quality data in CRM systems.
Outdated Data: Data containing old information due to lack of updates negatively impacts your marketing metrics and insights. Outdated data is even worse than duplicate data, because while the information might seem legitimate, it can up time and resources without you realizing. Knowing where your data originated from can help assess its
Strategies to Improve Data Quality
While poor quality data is a widespread issue, it is not an unsolvable one. Here are key strategies to clean up messy data and implement processes to maintain data integrity:
Implement Data Governance: A data governance framework formalizes data ownership, accountability, policies, and procedures around data management. This helps maintain data discipline.
Data Audits: First, you need to understand where your data quality pain points are. Audit your data regularly to identify outliers, inconsistencies, duplicates, and missing values. Use data profiling tools such as Anchor’s File View to help.
Continuous Data Cleanse: Dedicate resources to ongoing data cleansing initiatives to remove old, duplicate, incomplete, and inaccurate data on a regular basis. Don’t let data debt pile up.
Automate Data Validation: Using tools to automatically validate data according to your standardized rules helps catch and fix data quality issues in real-time before they get embedded in production systems.
Set Data Standards: Establish clear data standards around definitions, formats, requirements, and domains for each data field across the organization. Document and enforce these standards.
Train Your Team: Educate employees on data quality best practices, requirements, and the impact of poor data quality.
Monitor & Measure: Establish data quality metrics and keep tracking and reporting on them over time. You can’t manage what you don’t measure.
In Conclusion
By taking a proactive approach to data quality, organizations can turn one of their biggest struggles into a competitive advantage. The benefits of reliable, trustworthy data lead to smarter decision making, more efficient processes, improved ROI on marketing investments, and better experiences for employees and consumers.