In today’s data-driven business environment, the quality of data used for decision-making can make or break a company’s success. High-quality data is the foundation upon which informed decisions are made, strategies are crafted, and competitive advantages are gained.
This article explores the critical role of data quality in business decision-making, covering everything from data governance, tools, to best practices for maintaining data integrity.
Understanding Data Governance
Data governance is the framework that ensures data is managed as a valuable organizational asset. It encompasses the policies, procedures, and standards that control how data is collected, stored, processed, and used within an organization.
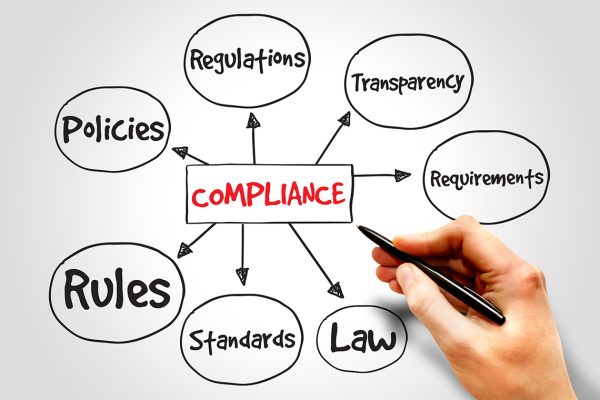
Effective data governance:
- Establishes clear ownership and accountability for data
- Ensures compliance with regulatory requirements
- Promotes consistent data usage across the organization
- Improves data quality and reliability
Characteristics of Quality Data
Quality data possesses 7 key characteristics:
- Accuracy: Data correctly represents the real-world entity or event it describes.
- Completeness: All necessary data is present without gaps, omissions, and errors.
- Consistency: Data is uniform across different systems and databases.
- Timeliness: Data is up-to-date and available when needed.
- Validity: Data conforms to defined formats, ranges, and rules.
- Uniqueness: No duplicates or redundant entries exist.
- Relevance: Data is appropriate and useful for the intended purpose
The Cost of Bad Data
According to Gartner’s most recent estimates, poor data quality costs organizations nearly $13 million annually. It can have severe consequences for businesses:
- Bad decisions: Inaccurate data can lead to poor decisions, resulting in revenue loss or increased expenses.
- Decreased productivity: Employees waste time dealing with data inconsistencies and errors.
- Damaged reputation: Incorrect information can harm customer relationships and brand image.
- Compliance risks: Inaccurate or incomplete data may lead to spam, regulatory violations, and penalties.
- Missed opportunities: Poor data quality can obscure valuable insights and market trends.
Several tools and technologies can help organizations maintain high data quality:
- Data profiling tools: Analyze data to uncover patterns, anomalies, and quality issues.
- Data hygiene: Automatically identify and correct errors in datasets.
- Suppression files: Deceased Suppression, MPS, Profanity, Prison, Nursing Homes, and APO/FPO.
- FileView: Anchor’s diagnostic tool that identifies the deliverability condition of data.
- ETL (Extract, Transform, Load) tools: Ensure data consistency when moving between systems.
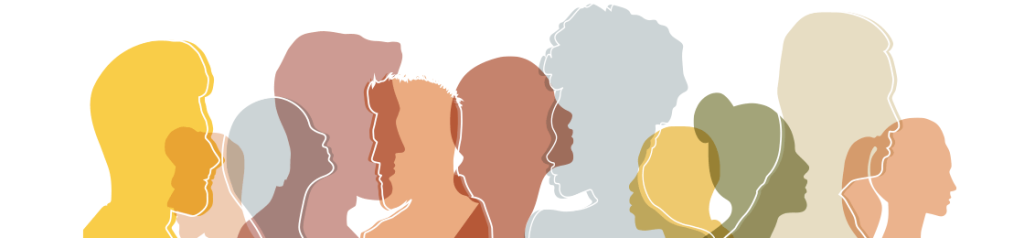
Consumer list profiling appends certain demographics to your list to create a consumer data profile. It helps to understand data structure, content, and relationships to identify patterns.
By regularly profiling data, organizations can proactively address quality issues and ensure that their data remains a reliable asset for decision-making.
Benefits of Quality Data
Investing in data quality yields many advantages:
- Improved decision-making and competitive advantage
- Increased efficiency with streamlined processes
- Enhanced customer satisfaction with better targeting and better service
- Reduced operational costs and mistakes
Best Practices for Ensuring Data Quality
To maintain high-quality data, organizations should follow these best practices:
- Implement a robust data governance program: Establish clear policies and procedures for data management.
- Conduct regular data audits: Periodically review and assess data quality across all systems and platforms.
- Train employees: Educate staff on the importance of data quality and proper data-handling procedures.
- Automate data quality checks: Use tools to continuously monitor and flag data issues.
- Standardize data entry processes: Create consistent guidelines for data input to reduce errors at the source.
- Implement data validation rules: Set up checks to ensure data meets predefined quality criteria before entering systems.
- Establish a data quality culture: Make data quality a priority across all levels of the organization.
The importance of data quality in business decisions cannot be overstated. According to McKinsey, 60% of tech executives highlight poor data quality as the primary roadblock to scaling their data-driven operations. By investing in data governance, leveraging appropriate tools, and following best practices, organizations can ensure that their data is accurate, consistent, and dependable.
Get to know more about how we help and when you’re ready to get started, drop us a line 800-452-2357.